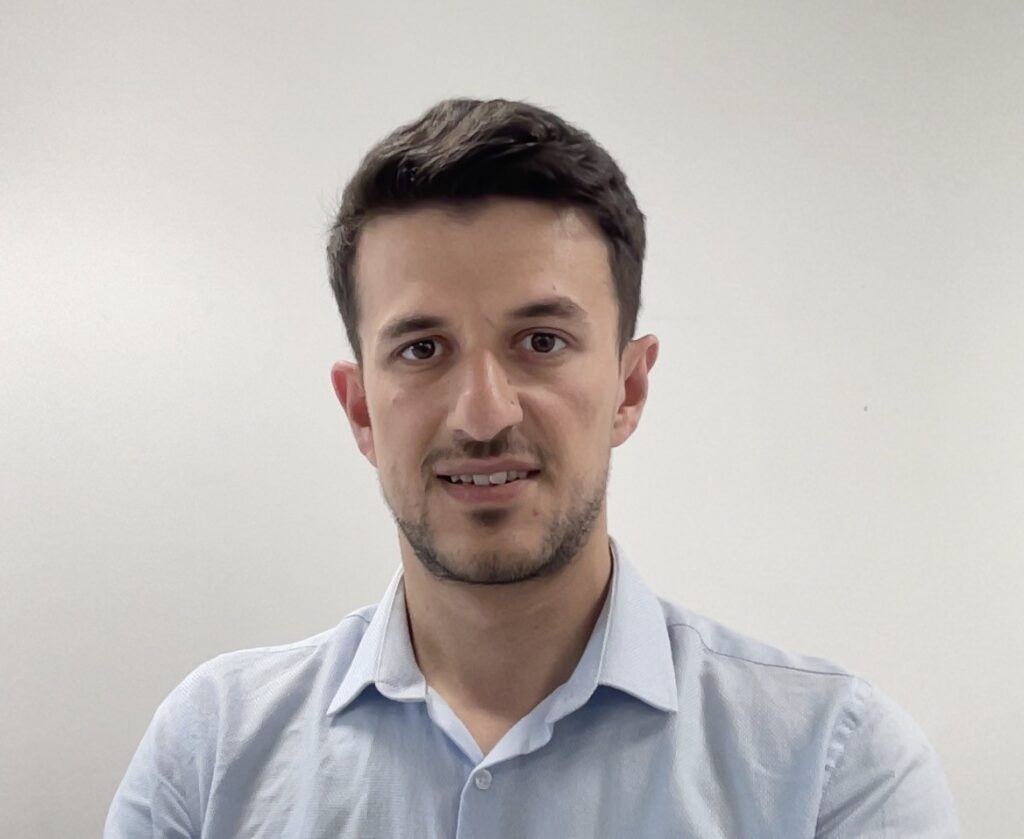
Mohammad Fattahi Sani (Moe Sani)
Mohammad Fattahi Sani (Moe Sani) is currently working as Associate Principal Software Engineer at Dyson‘s Robotics department. He and his team are building intelligent robot software and behaviours for next generation of Dyson’s robots.
Moe has also been serving as Industrial Mentor for Dyson Institute of Technology (DIET) as well as University of Bristol students since 2020.
Prior to Dyson, Moe was a Research Associate at Bristol Robotics Laboratory (BRL), working on SMARTsurg European project to build a more intuitive teleoperated surgical robot. During his research at BRL, he contributed to different aspects of the project including hand tracking, teleoperation, virtual reality and haptic feedback.
Moe has also worked as a Fellow at Italian Institute of Technology(IIT)’s Advanced Robotics Lab (ADVR) where he was part of a cutting edge projects called The Robot Teleoperativo project, which aims for substituting humans in hazardous environments with a quadruped robotic platform operated by an immersive virtual reality master station. Moe was a member of the user interface group where he successfully contributed to many aspects of the project including Sound Source Localisation (SSL), and telemetry aspects. He designed and implemented a novel approach in sound source position estimation which can be helpful in efficiently identifying the 3D location of the fire or earthquake accident victims by a moving robot that carries a microphone array. The outcome of this impactful research has been presented and published in the 19th International Conference on Advanced Robotics (ICAR) in Brazil.
Before IIT, Moe was a member of R&D department at Sobh Parlar Industrial Co. where he delivered several products in the field of household appliances.
Selected Publications
- Mapping Surgeons Hand/Finger Movements to Surgical Tool Motion During Conventional Microsurgery Using Machine Learning
- Automatic navigation and landing of an indoor AR. drone quadrotor using ArUco marker and inertial sensors
- Experimental study of reinforcement learning in mobile robots through spiking architecture of Thalamo-Cortico-Thalamic circuitry of mammalian brain
- Towards finger motion tracking and analyses for cardiac surgery
- Unsupervised learning of target attraction for robots through spike timing dependent plasticity
- Palm Reading: Using Palm Deformation for Fingers and Thumb Pose Estimation
- Towards Sound-source Position Estimation using Mutual Information for Next Best View Motion Planning